Can Winning A/B Tests Be Predicted With Past Results?
For any professional to actually deserve such a designation, their promised outcomes should at the very least perform a tiny bit better than randomness. So if we're in the business of recommending design changes or design experiments, more than half of those recommendations ought to carry their predicted positive impact. Otherwise, we're as good as charlatans helping and hurting others equally by chance alone. This bring me to a question: can we increase our prediction rate of positive or negative experiment outcomes by remembering and using past a/b tests?
What's Your Opinion?
My Opinion (With Data) :)
Clearly my own view is such that generalizable UI patterns do exist among an infinite pool of ideas. To be fully transparent I'd also like to admit that I do have income from providing access to such evidence-based patterns along with their varying success rates (including those with flat or negative effects).
Acknowledging Many Low Quality Experiments
First of all, let's acknowledge that a lot of mistrust towards online published a/b tests is rightfully justified. As experimentation started becoming popular, people rushed to share their big winners such as +21% and +76% conversion gains from trust seals. Many of such test are rooted in low statistical power with overly optimistic and inflated effects that often come down given enough time (from higher statistical power). More so, this problem is further compounded by the human bias to publish positive results more so than negative or insignificant ones. Both of these problems have undermined trust by making predictions more difficult.
One solution that we take is to run experiments longer, compare multiple experiments together and publish all results independent of their outcomes. By aiming for more honesty, predicted effects of given patterns tend to become more accurate. In some cases, that also means that some patterns such as trust seals begin to show very little or flat expected effects (based on 4 similar experiment results from our own data).

Developing An Appetite For Better Predictions
I've first experienced the satisfaction of copying (predicting) experiments while running an optimization business - guiding companies on what to a/b test. It's inevitable that as we ran tests some successes were cross-pollinated, copied, or replicated (whatever you call it) between different clients. And when a success for one client worked for another, it felt ... great. Successful replication of experiments deepens the sense that there is some order in the universe. :) It means that stuff isn't just behaving randomly. It means that we are learning. And it means we can share the knowledge with others and increase their odds of success (or learn from their failed replication attempts - which is also highly valuable).
The Big Question: Can Winning A/B Tests Be Predicted With Past Results?
Last year we made our first attempt to actually crunch some numbers and begin answering the big question - can winning a/b tests be predicted with past results? In order to assess if patterns have any improved predictive power, we began tracking our own predictions across numerous optimization projects. We ran and tracked 51 such a/b tests that were strictly pattern driven and here are the exciting results we found.
Out of 51 experiments which were all predicted to be positive with varying degrees of repeatability (a net count of positive - negative experiments to gauge how often a patterns tends to win or lose), 36 of these experiments ended being positive at the time of stopping. This placed the prediction rate of these patterns at 71% - helping us do better than 50/50. This initial measure provided us with a very positive outlook towards evidence-based patterns as an effective way in running more winning a/b tests than chance alone.
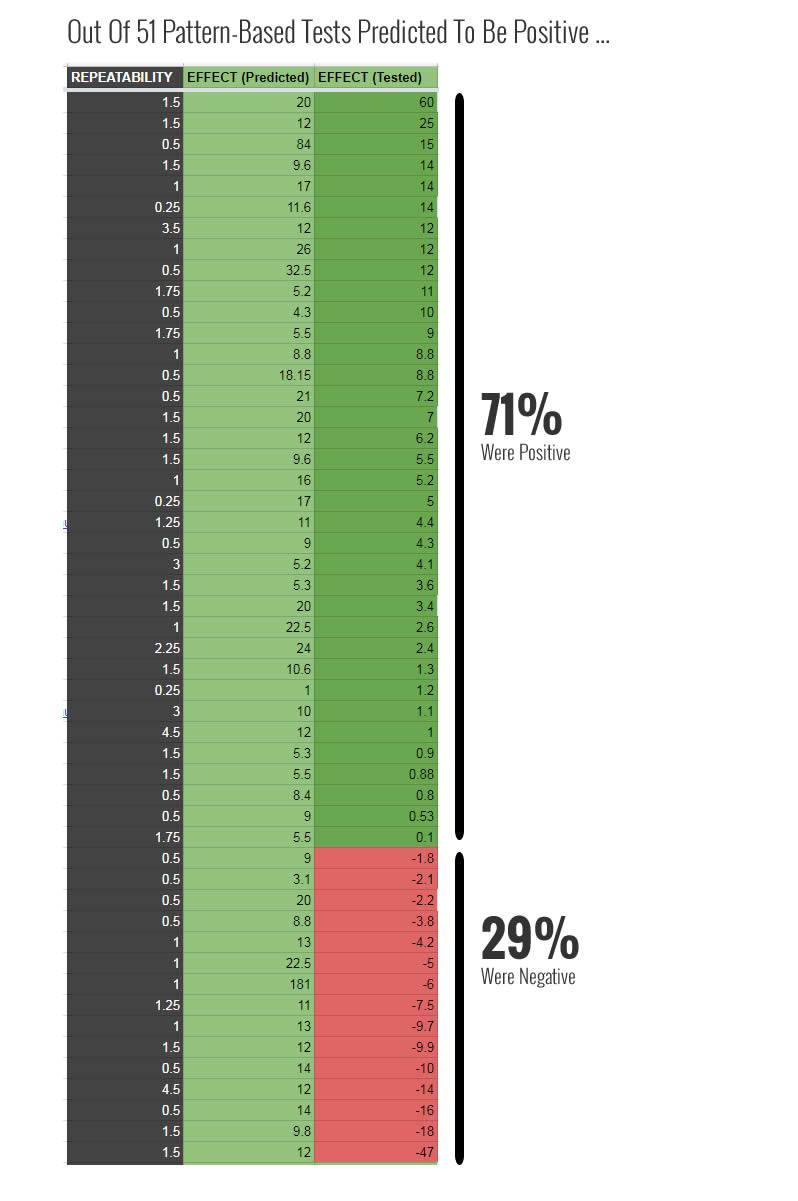
The Bigger Question: Can More Winning A/B Tests Be Predicted With More Repeatable Results?
There is one more key question that we can ask ourselves in order to check if repeatability is a reliable predictor of test outcomes: does a higher repeatability score lead to more positive test predictions? In other words, if a pattern performs positively more frequently, does that mean that it has better chances of performing positively in future experiments? To answer this question we organized our predictions by three sets of repeatability scores (reminder: the higher the score, the more positive evidence we have in favor of a pattern). Here is what we found:
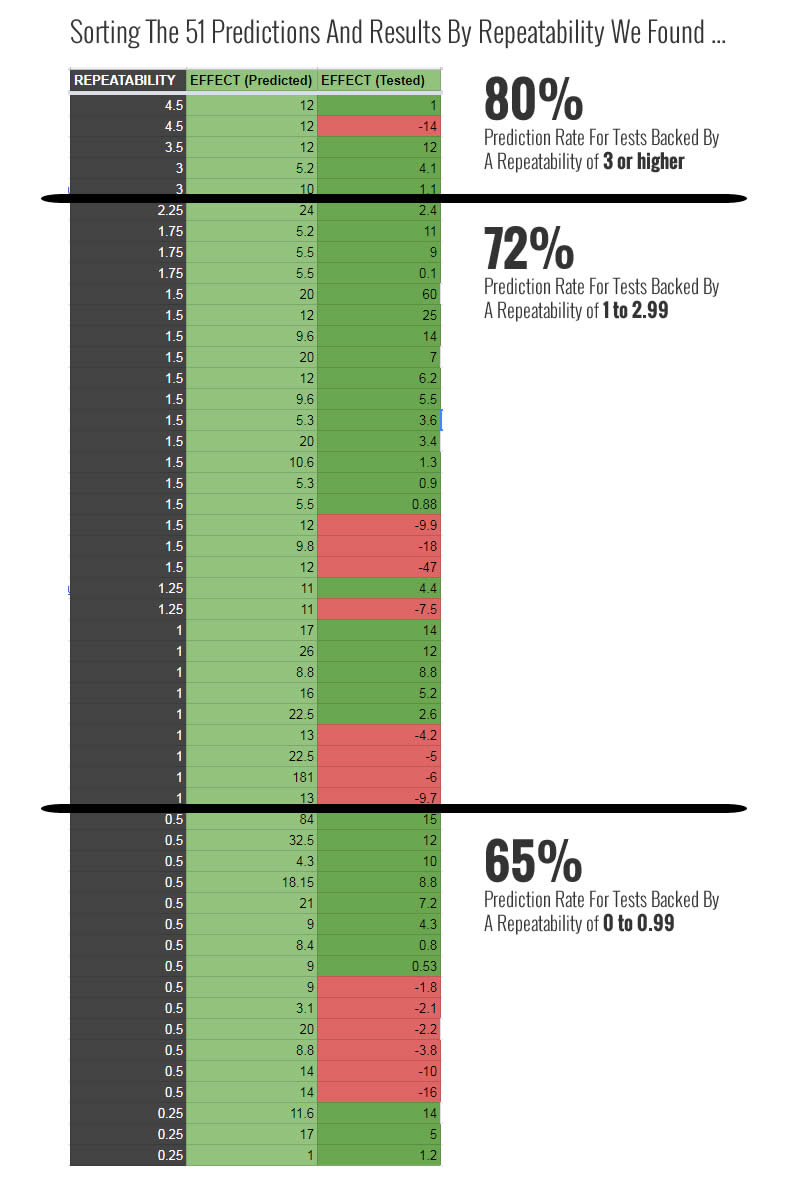
If true, this second chart might be supporting the idea that "the more a pattern has worked in the past, the more likely it will also work again in the future" - the predictive power of reproducbility in a nutshell.
The Ignorance-Arrogance Spectrum
When making predictions there is one last thing to keep in mind. On one end of the spectrum, some people might absolutely believe that they are blank slates, they shouldn't assume (predict) anything, and that any existing evidence should not be trusted as a source of future outcomes. This extreme end we might call ignorance.
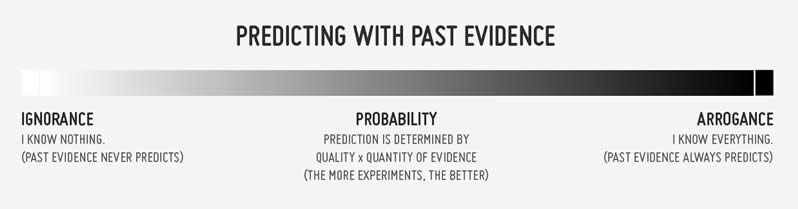
On the other end of the predictive spectrum, we might see some other people giving absolute weight to any evidence they come across. Whenever such people see an a/b test, they might refer to it as ultimate proof. This we can call arrogance.
In the middle are people who see past experiment results as probabilities. The higher the quality and quantity of stacking evidence, the more weight is assigned to the evidence. And this is how I view and encourage others to use past results when predicting future outcomes.
What's Your Take On Predicting A/B Tests?
Please share your thoughts, experiences and data. :)
Comments
Ondřej Ilinčev 6 years ago ↑0↓0
I would argue that there is not a 50/50 chance of success of a random change on the web. Most changes do not make any difference. VWO ran an analysis of all past A/B tests and put the success ratio at 1 in 8 (significance 95%, positive outcome).
Reply
Jakub Linowski 6 years ago ↑0↓0
Hi Ondřej. There might be a big difference between what constitutes a winning test (higher and custom standard) vs whether simply the test was positive or negative. We looked at the latter because all companies may have their own standards or thresholds for acceptance.
The other thing to keep in mind when companies like VWO (tools) or Google (high velocity testing teams) report such success rates, is that there might be multiple variables in play. These could include:
1. Experience level (+ more experienced teams should have higher win rates)
2. Degree of optimization (- more optimized sites should see lower win rates)
3. Traffic & testing power (- lower traffic sites should detect fewer winners)
4. Prior probability (+ tests based on past successes should see higher win rates - if my analysis above is correct)
Reply
Ondřej Ilinčev 6 years ago ↑1↓0
True. What I am trying to say is that most optimizers would kill for a 50% success rate.
Reply
Jakub Linowski 6 years ago ↑1↓0
Ondrej, this is my goal for GoodUI - to increase your odds of getting there - at least in the next 10 experiments you run
Reply
Kevin Anderson 6 years ago ↑0↓0
Hi Jakub, great analysis you did here. I will include it in my weekly newsletter about analytics & experimentation: Behavioral Insights.
Reply